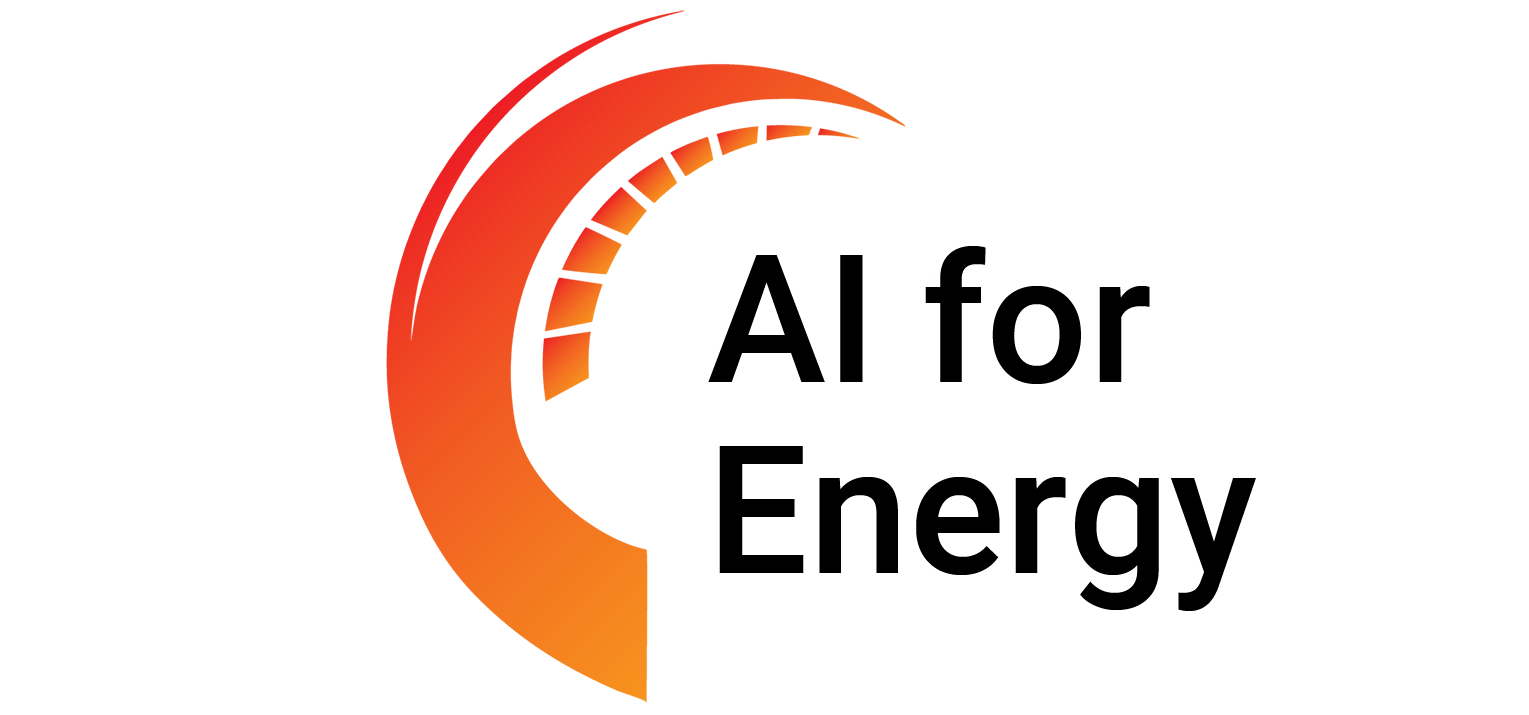
The end-use case in energy systems tackles a fundamental societal challenges: how to power the electricity grid with a vast majority of renewable energy resources such as solar and wind farms, and hydropower. These renewable resources introduce significant stochasticity in front and behind the meter and increase forecasting errors. Their availabilities are also not necessarily aligned, temporally and spatially, with the demand, creating the need for storage solutions and demand response programs. These challenges will be further exacerbated by the anticipated electrification of the transportation system.
The Institute will explore forecasting, machine-learning, and optimization methods to solve the resulting planning and operational challenges. It studies both how to enhance existing market-clearing algorithms to accommodate the induced stochasticity and manage risks. It also develops new decentralized control and optimization algorithms to accommodate the massive increase in distributed energy resources. This end use case will collaborate closely with the end-to-end learning and optimization, distributed optimization, and decision making under uncertainty thrusts.
Some of the contributions of this end-use case include
The Just-In-Time Learning methodology which trains machine-learning models for the security-constrained economic dispatch under different generator commitment.
Self-supervised learning for constrained optimization which learns both primal and dual values in an augmented Lagrangian framework for applications in power systems.
The combination of confidence-aware graph-neural networks and fast feasibility restoration to guarantee feasibility in reliability commitment problems (FRAC and LAC in MISO terminology).
A novel methodology to generate high fidelity time series for power systems test cases.
The compact optimization learning methodology that learns OPF problems with up to 30,000 buses.
Warm-starting ADMM Algorithms with optimal primal and dual estimates for learning decentralized power systems.
A short presentation of the RAMC project articulates some of the motivations and approaches that seeded this end-use case.