Self-Supervised Primal-Dual Learning for Constrained Optimization. Seonho Park and Pascal Van Hentenryck. In the Thirty-Seven AAAI Conference on Artificial Intelligence (AAAI-23), February 2023.
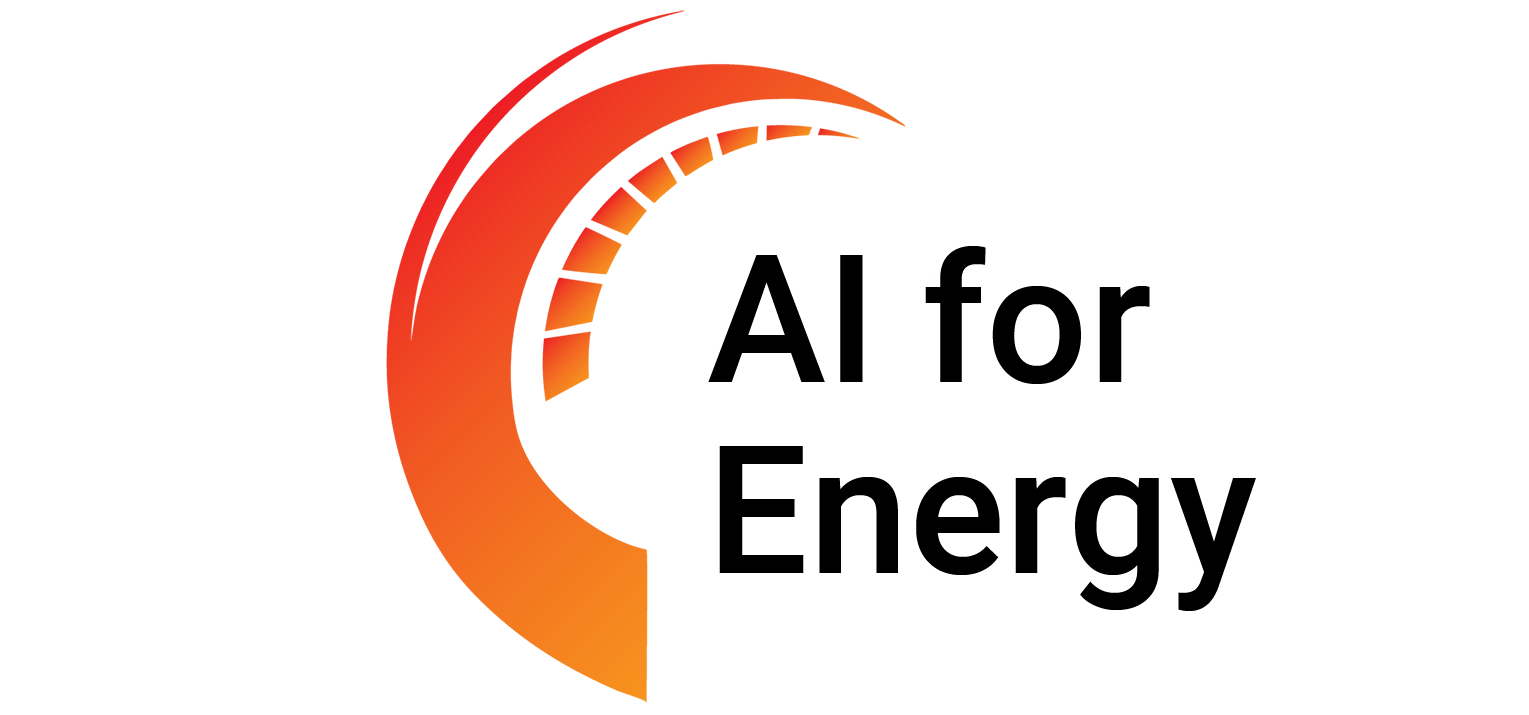
AI for Energy
The end-use case in energy systems tackles a fundamental societal challenges: how to power the electricity grid with a vast majority of renewable energy resources such as solar and wind farms, and hydropower. These renewable resources introduce significant stochasticity in front and behind the meter and increase forecasting errors. Their availabilities are also not necessarily aligned, temporally and spatially, with the demand, creating the need for storage solutions and demand response programs. These challenges will be further exacerbated by the anticipated electrification of the transportation system.
The Institute will explore forecasting, machine-learning, and optimization methods to solve the resulting planning and operational challenges. It studies both how to enhance existing market-clearing algorithms to accommodate the induced stochasticity and manage risks. It also develops new decentralized control and optimization algorithms to accommodate the massive increase in distributed energy resources. This end use case will collaborate closely with the end-to-end learning and optimization, distributed optimization, and decision making under uncertainty thrusts.
Some of the contributions of this end-use case include
- The Just-In-Time Learning methodology which trains machine-learning models for the security-constrained economic dispatch under different generator commitment.
- Self-supervised learning for constrained optimization which learns both primal and dual values in an augmented Lagrangian framework for applications in power systems.
- The combination of confidence-aware graph-neural networks and fast feasibility restoration to guarantee feasibility in reliability commitment problems (FRAC and LAC in MISO terminology).
- A novel methodology to generate high fidelity time series for power systems test cases.
- The compact optimization learning methodology that learns OPF problems with up to 30,000 buses.
- Warm-starting ADMM Algorithms with optimal primal and dual estimates for learning decentralized power systems.
A short presentation of the RAMC project articulates some of the motivations and approaches that seeded this end-use case.
Publications
Funded by the Institute
-
Learning Regionally Decentralized AC Optimal Power Flows with ADMM, Terrence Mak, Minas Chatzos, Mathieu Tanneau, and Pascal Van Hentenryck. IEEE Transactions on Smart Grids, 14(6), 4863-4876. November 2023.
-
End-to-End Feasible Optimization Proxies for Large-Scale Economic Dispatch. Wenbo Chen, Mathieu Tanneau, and Pascal Van Hentenryck. IEEE Transactions on Power Systems (to appear).
-
Compact Optimization Learning for AC Optimal Power Flow, Seonho Park, Wenbo Chen, Terrence W.K. Mak, and Pascal Van Hentenryck, IEEE Transactions on Power Systems (to appear).
-
Load Embeddings for Scalable AC-OPF Learning. Terrence W.K. Mak, Ferdinando Fioretto, and Pascal Van Hentenryck. 2023 IEEE Power & Energy Society (PES) General Meeting, Orlando, Florida, July 2023.
-
Confidence-Aware Graph Neural Networks for Learning Reliability Assessment Commitments. Seonho Park, Wenbo Chen, Dahye Han, Mathieu Tanneau, and Pascal Van Hentenryck. IEEE Transactions on Power Systems (to appear).
-
-
Learning Optimization Proxies for Large-Scale Security-Constrained Economic Dispatch. Wenbo Chen, Seonho Park, Mathieu Tanneau, and Pascal Van Hentenryck. Electric Power Systems Research, Volume 213, December 2022, 108566.
-
Learning Optimization Proxies for Large-Scale Security-Constrained Economic Dispatch. Wenbo Chen, Seonho Park, Mathieu Tanneau, and Pascal Van Hentenryck. In the Proceedings of the 22nd Power Systems Computation Conference (PSCC), June 27 – July 1, 2022 in Porto, Portugal.
-
A Linear Outer Approximation of Line Losses for DC-based Optimal Power Flow Problems. Haoruo Zhao, Mathieu Tanneau, and Pascal Van Hentenryck. In the Proceedings of the 22nd Power Systems Computation Conference (PSCC), June 27 – July 1, 2022 in Porto, Portugal.
-
Data-Driven Time Series Reconstruction for Modern Power Systems Research. Minas Chatzos, Mathieu Tanneau, and Pascal Van Hentenryck.Data-Driven Time Series Reconstruction for Modern Power Systems Research. In the Proceedings of the 22nd Power Systems Computation Conference (PSCC), June 27 – July 1, 2022 in Porto, Portugal.
-
Risk-Aware Control and Optimization for High-Renewable Power Grids. Neil Barry, Minas Chatzos, Wenbo Chen, Dahye Han, Chaofan Huang, Roshan Joseph, Michael Klamkin, Seonho Park, Mathieu Tanneau, Pascal Van Hentenryck, Shangkun Wang, Hanyu Zhang, Haoruo Zhao. arXiv:2204.00950, April 2022.
Related work
-
Spatial Network Decomposition for Fast and Scalable AC-OPF Learning.Minas Chatzos, Terrence Mak, and Pascal Van Hentenryck. IEEE Transactions on Power Systems, 37(4), 2601--2612, July 2022.
-
Spatial Network Decomposition for Fast and Scalable AC-OPF Learning. Minas Chatzos, Terrence Mak, and Pascal Van Hentenryck. IEEE Transactions on Power Systems (Early access: 10.1109/TPWRS.2021.3124726).
-
Machine Learning for Optimal Power Flows. Pascal Van Hentenryck. INFORMS TutORials 2021.
-
Alexandre Velloso, Pascal Van Hentenryck, and Emma Johnson. An Exact and Scalable Problem Decomposition for Security-Constrained Optimal Power Flow. Electric Power Systems Research, (to appear).
-
Combining Deep Learning and Optimization for Preventive Security-Constrained DC Optimal PowerFlow.Alexandre Velloso and Pascal Van Hentenryck. IEEE Transactions on Power Systems, 36(4), July 2021.
-
Fair and Reliable Reconnections for Temporary Disruptions in Electric Distribution Networks using Submodularity, with Cyrus Hettle, Daniel Molzahn, under submission 2021. Preprint available at arxiv: https://arxiv.org/abs/2104.07631.
-
Communication-Constrained Expansion Planning for Resilient Distribution Systems. Geunyeong Byeon, Pascal Van Hentenryck, Russell Bent, Harsha Nagarajan. INFORMS Journal on Computing, 32(4): 968-985, 2020.
-
Unit Commitment with Gas Network Awareness. Geunyeong Byeon and Pascal Van Hentenryck. IEEE Transactions on Power Systems, 35(2), 1327–1339, March 2020.
-
Predicting AC optimal power flows: Combining deep learning and lagrangian dual methods. Ferdinando Fioretto, Terrence W.K. Mak, and Pascal Van Hentenryck. Proceedings of the AAAI Conference on Artificial Intelligence, 34(01):630–637, 2020.
-
A bound strengthening method for optimal transmission switching in power systems. S. Fattahi, J. Lavaei, and A. Atamturk. IEEE Transactions on Power Systems, 34(1):280–291, 2019.