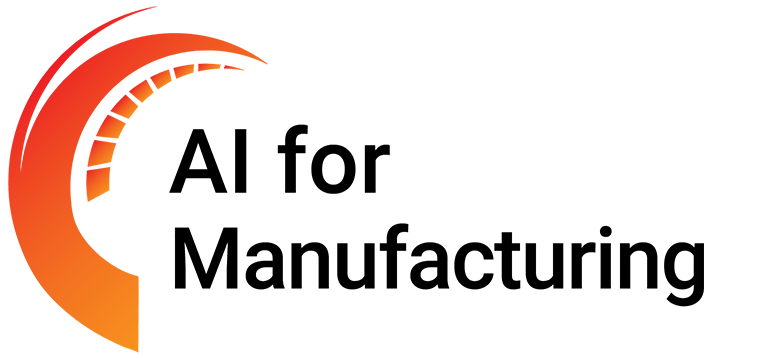
The U.S. National Science Foundation AI Research Institute for Advances in Optimization (NSF AI4OPT) works with manufacturing partners on some critical challenges. These challenges range from the lack of synchronization between supply chains and manufacturing systems, the need for more resilient and adaptive manufacturing lines, the sheer size of modern manufacturing systems that involve tens of millions of decisions, the complex scheduling problems at the core of manufacturing processes, to the design process themselves. NSF AI4OPT collaborates widely in this area with industries in the semi-conductor fabrication to packaging and surfaces.