Bistra Dilkina, associate professor of computer science at the University of Southern California and co-director of the USC Center for AI in Society, delivered a seminar titled "The Effective Synergy of Machine Learning and Combinatorial Solvers." The presentation focused on integrating machine learning techniques with combinatorial optimization to enhance solver performance.
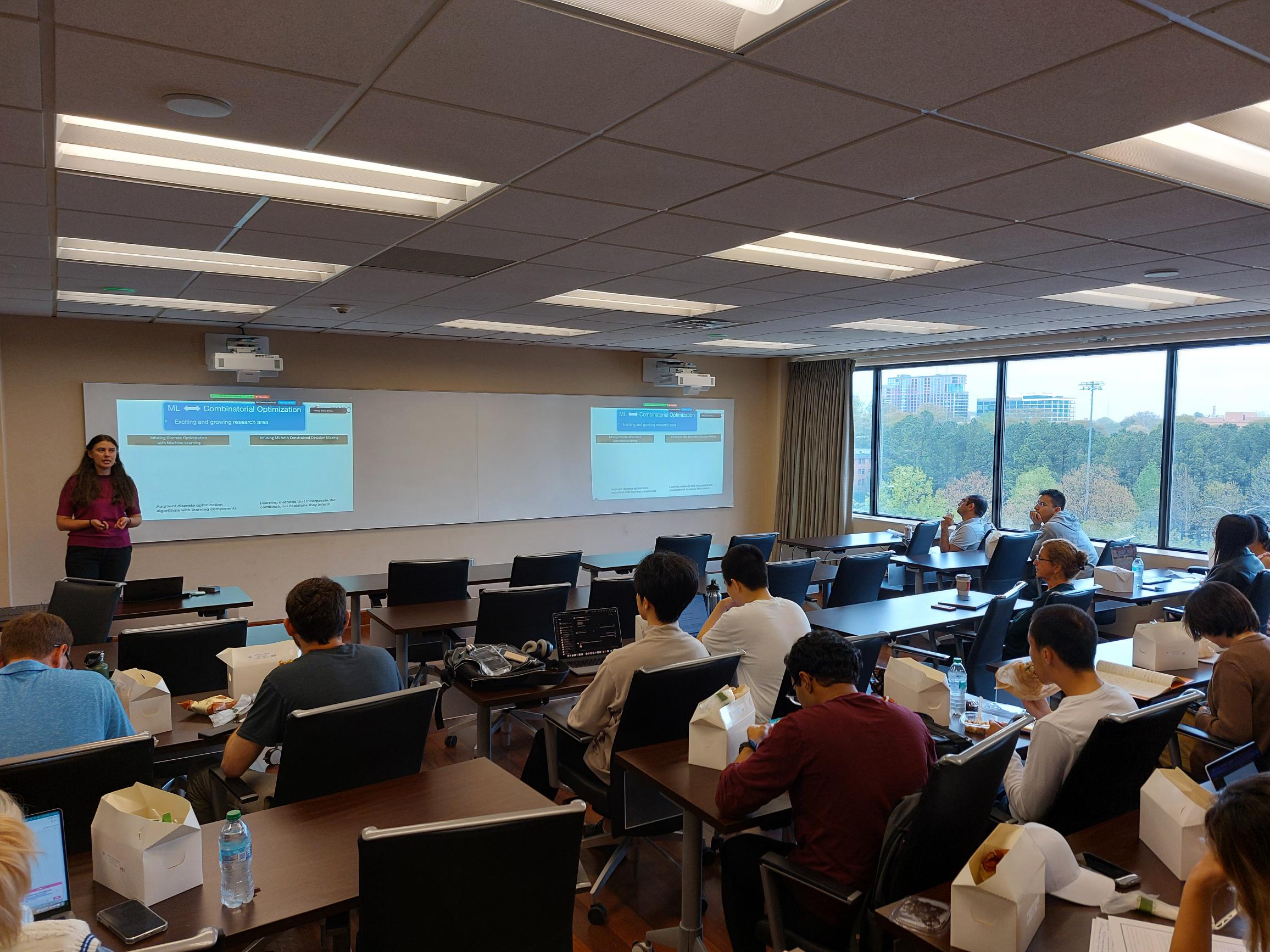
Integrating Machine Learning with MILP Solvers
Dilkina discussed recent advancements in augmenting Mixed Integer Linear Programming (MILP) solvers with machine learning-guided components. By leveraging historical data from similar problem instances, these integrations aim to improve both the speed and quality of solutions. She highlighted the application of contrastive loss in tasks such as Large Neighborhood Search and backdoor selection within MILP, demonstrating significant performance enhancements across multiple domains.
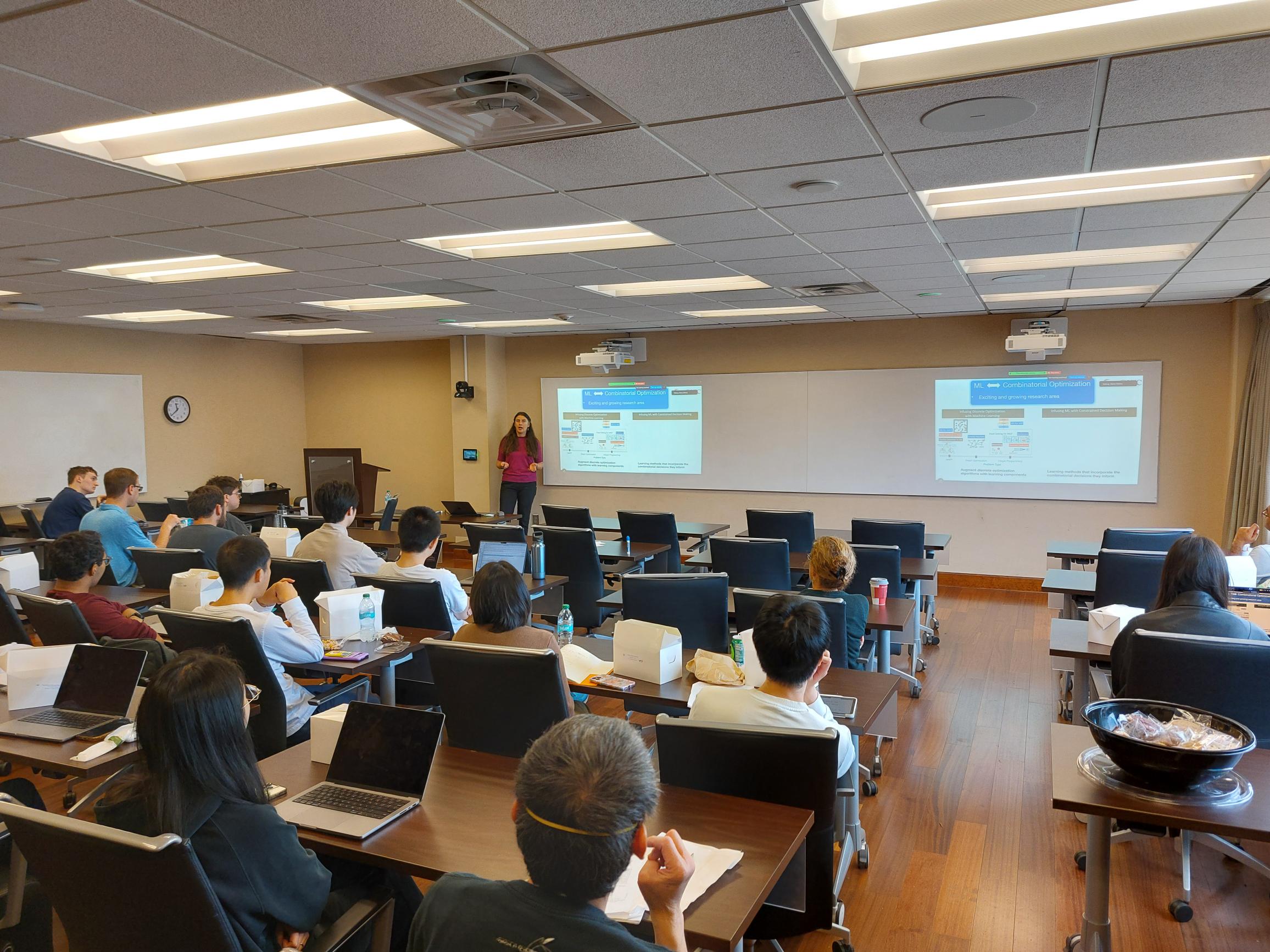
Introducing d-MIPLIB for Benchmarking
To support the research community, Dilkina introduced d-MIPLIB (Distributional MIPLIB), a unified benchmark set designed to facilitate evaluation across various problem domains. This resource aims to drive progress in machine learning-guided MILP solving by providing a standardized platform for comparison and analysis.
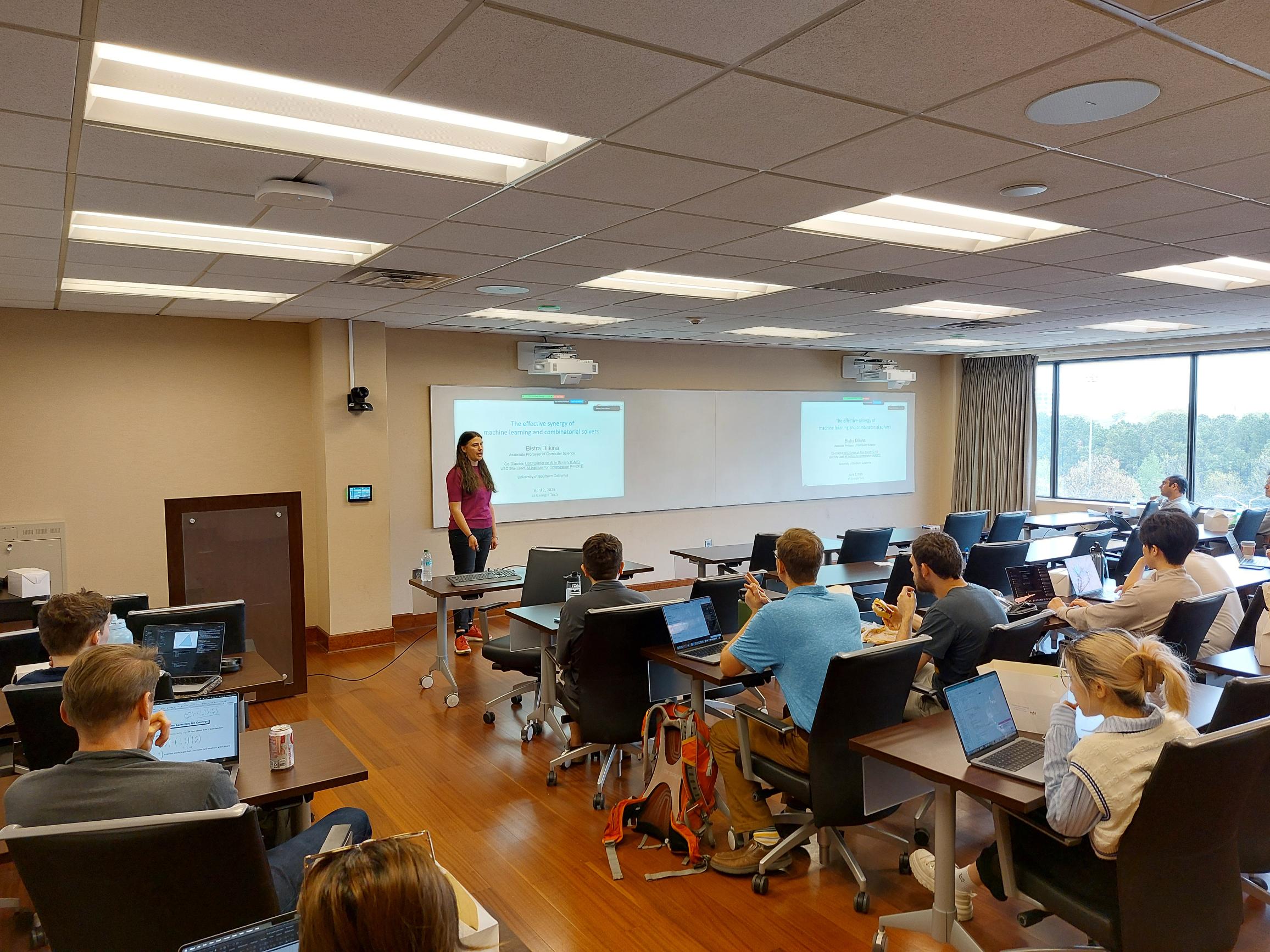
Potential of Multi-Task Learning
The seminar also explored the potential of multi-task learning across different machine learning-guided solving techniques. Dilkina emphasized how learning across multiple domains can be particularly beneficial when historical examples are limited, setting the stage for foundational models in ML-guided MILP solving.
Watch the seminar here.