Our featured researchers are at the forefront of addressing pressing challenges in energy, supply chain management, sustainability, and chip design challenges while fostering education initiatives.
Explore their incredible contributions
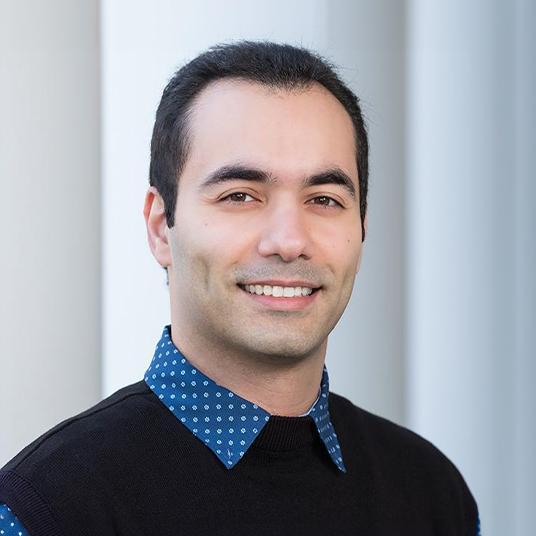
Vahid Eghbal Akhlaghi
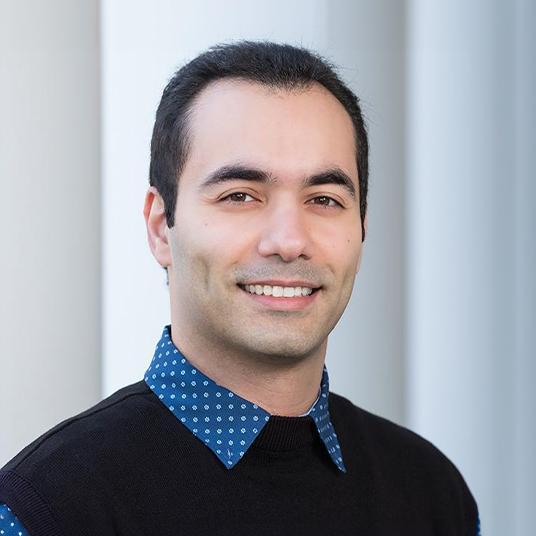
Addressing Today's Challenges and Tomorrow's Opportunities in Optimizing Supply Chains
Abstract: We spoke with Vahid Eghbal Akhlaghi, a member of the AI Institute for Advances in Optimization (AI4OPT) and summarized our conversation about how AI and optimization are being used to tackle key challenges facing supply chains. One of the biggest challenges facing supply chains today is the complexity of operations involving various people and processes. Additionally, issues such as political instability, trade conflicts, supplier problems, and natural disasters like the Covid-19 pandemic can lead to delays and increased costs. Sustainability is another concern, with a focus on reducing waste, carbon emissions, and environmental harm.
"AI4OPT is developing advanced decision-making tools and algorithms that leverage AI and optimization techniques to address these challenges," said Akhlaghi. "By using AI algorithms, businesses can improve their supply chain operations in several ways: Optimizing inventory management by analyzing historical demand patterns, current inventory levels, and lead times to predict future demand and adjust inventory levels accordingly." Optimizing transportation routes, warehouse operations, fleet performance, and transportation mode selection to reduce costs, improve efficiency, and enhance customer satisfaction. Identifying potential risks in the supply chain to minimize disruptions and improve resilience. Forecasting demand by analyzing large datasets and identifying patterns that traditional methods may overlook. Helping companies meet sustainability goals by optimizing supply chain operations and reducing environmental impact. "AI4OPT aims to continue advancing algorithms and solutions for more substantial and complex optimization problems using AI," added Akhlaghi about the institute which also prioritizes ethical AI use, transparency, fairness, privacy, sustainability, collaboration, accountability, and human control in supply chain management to ensure the interests of all stakeholders are protected.
Biography: Vahid Eghbal Akhlaghi is a postdoctoral fellow for the AI Institute for Advances in Optimization (AI4OPT). He earned his Ph.D. in Business Analytics from the University of Iowa, where he utilized operations research methodologies to solve logistical challenges related to disaster preparedness and recovery. He currently serves as Chief of Staff for the AI4OPT supply chain projects at Georgia Tech, where he provides strategic leadership and technical expertise in overseeing and contributing to various supply chains, transportation, and scheduling projects.
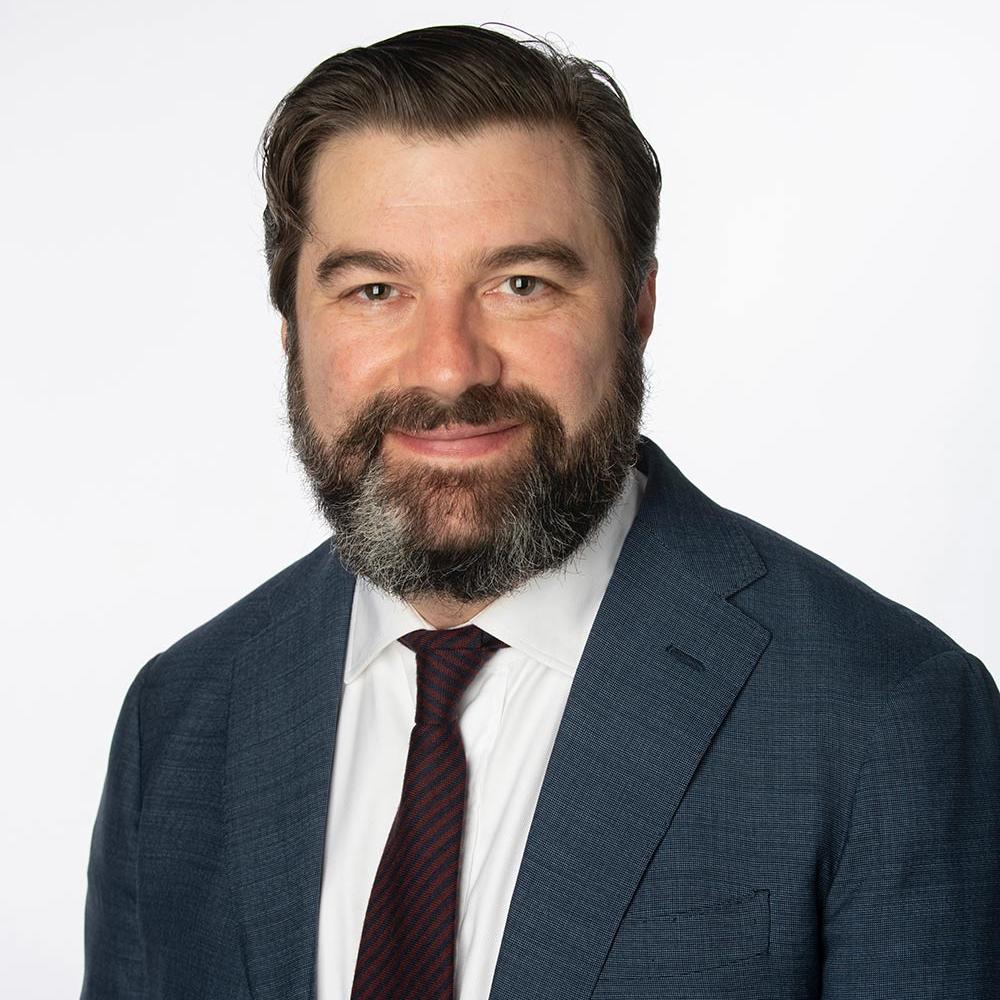
Justin Biddle
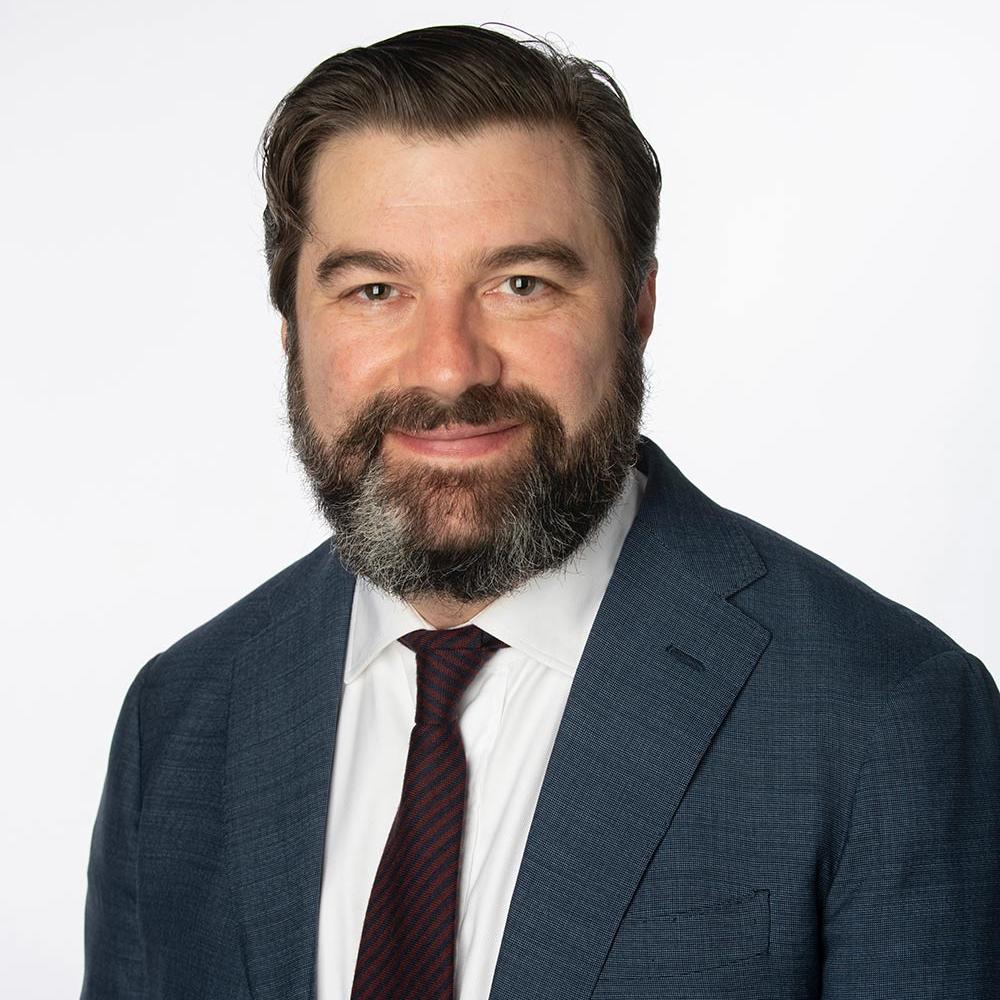
Justin Biddle
Abstract: My background is in the philosophy of science and technology and the ethics of emerging technologies. I’m particularly interested in artificial intelligence and ethical issues in machine learning. The most common approaches to AI ethics are principle based. Many AI companies and organizations are publishing AI ethics codes that highlight high-level principles like responsibility, fairness, and accountability, which are supposed to guide the design of AI systems. The consideration of these principles should reduce bias, prevent discrimination, and lead to benefits for humanity. But, while these principles are valuable, it’s often not clear how organizations interpret these principles and apply them in specific cases. How effective are these principals at improving the design of AI systems?
Some of the work I am doing with Swati Gupta (lead of AI4OPT’s Ethical AI and assistant professor and Fouts Family Early Career professor in the H. Milton Stewart School of Industrial & Systems Engineering) and others is identifying practices for designing AI systems in an ethical and responsible way – practices that structure the kinds of questions researchers are asking and whom they are engaging. The Institute of Electrical and Electronics Engineers (IEEE), the world's largest technical professional organization dedicated to advancing technology for the benefit of humanity, has put out a standard that incorporates practices and processes (not just principles) for ethical and responsible design. A paper we are currently working on will show ways in which the IEEE Standard can be adapted into AI4OPT. Though the IEEE standard focuses on AI generally, we think that it can be refined for what AI4OPT is looking to achieve.
This standard focuses on identifying and understanding the ways in which an AI system might impact different dimensions of human well-being. Oftentimes, systems are developed to promote some aspects of well-being (e.g., economic benefits for producers that result from efficiency gains), and other dimensions of well-being (e.g., physical or mental health of workers) can be neglected. There are often unintended consequences of AI systems, which can impact different dimensions of well-being. This standard attempts to identify the variety of different dimensions of well-being that are impacted by an AI system, and to articulate ways in which we can gather data that will illuminate these different well-being impacts. Ideally, this standard can help us create a “well-being indicators dashboard” that can be used by researchers to shed light on these impacts and adjust the AI system to better promote human well-being.
A well-being indicators dashboard is created in two phases:
- The first phase is internal, where researchers themselves try to answer a set of questions about what the AI system does, who might be impacted by it, what the risks and benefits might be for different stakeholders, and so on.
- The second phase builds on the first by identifying potential stakeholders and engaging them about how the system might impact them. This phase is important, because researchers and designers of AI systems do not necessarily understand the concerns and experiences of those impacted by the systems they are creating.
Both phases can help to better understand how AI systems impact well-being in its variety of dimensions, and this knowledge can in turn be used to redesign the systems to better promote human well-being.
I am motivated to address the vagueness of many AI ethics codes by identifying, implementing, and assessing standards for ethical and responsible design of AI systems, such as the one produced by IEEE. Standards like this can hopefully guide data scientists and researchers to build AI systems in an ethical manner that benefit society.
Biography: Justin Biddle co-leads AI4OPT’s Ethical AI thrust. He is also an associate professor in the School of Public Policy at the Georgia Tech and director of the new Ethics, Technology and Human Interaction Center (ETHICx). Biddle’s research interests are in philosophy of science and technology, ethics of emerging technologies (especially AI), and science and technology policy.
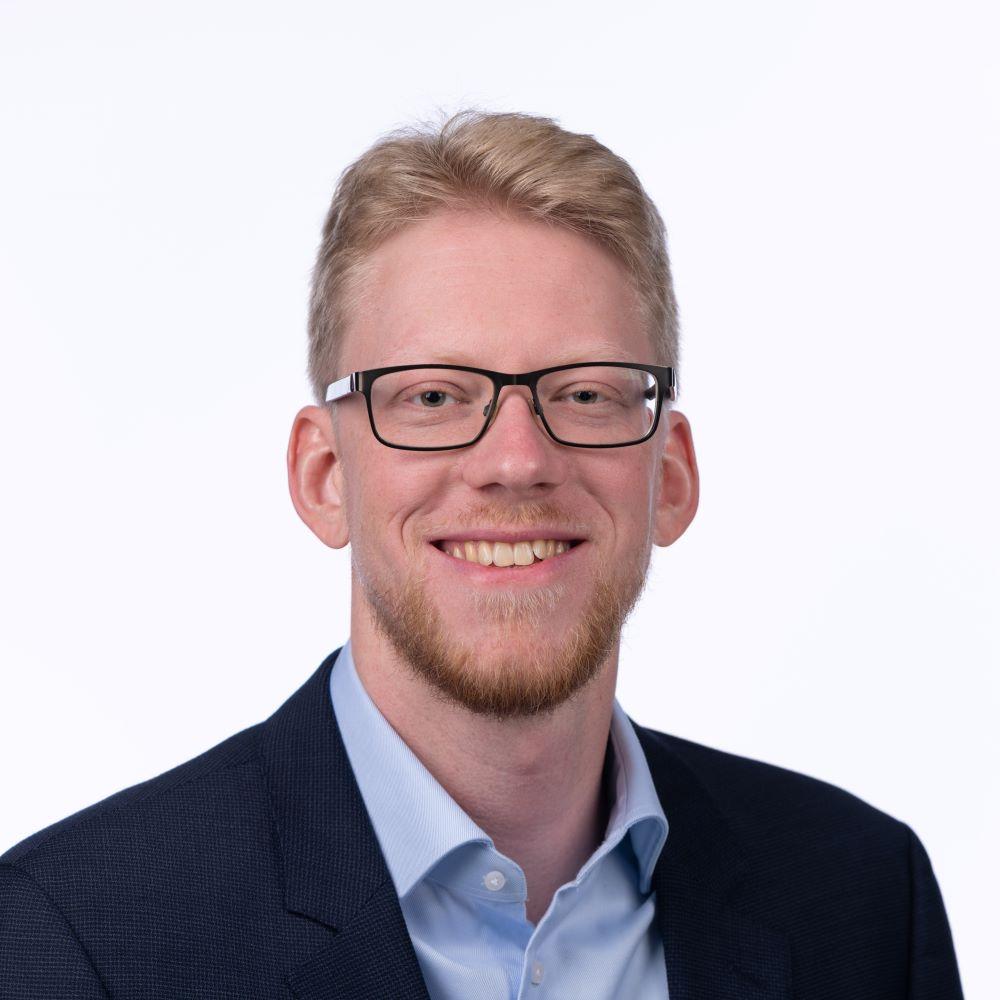
Kevin Dalmeijer
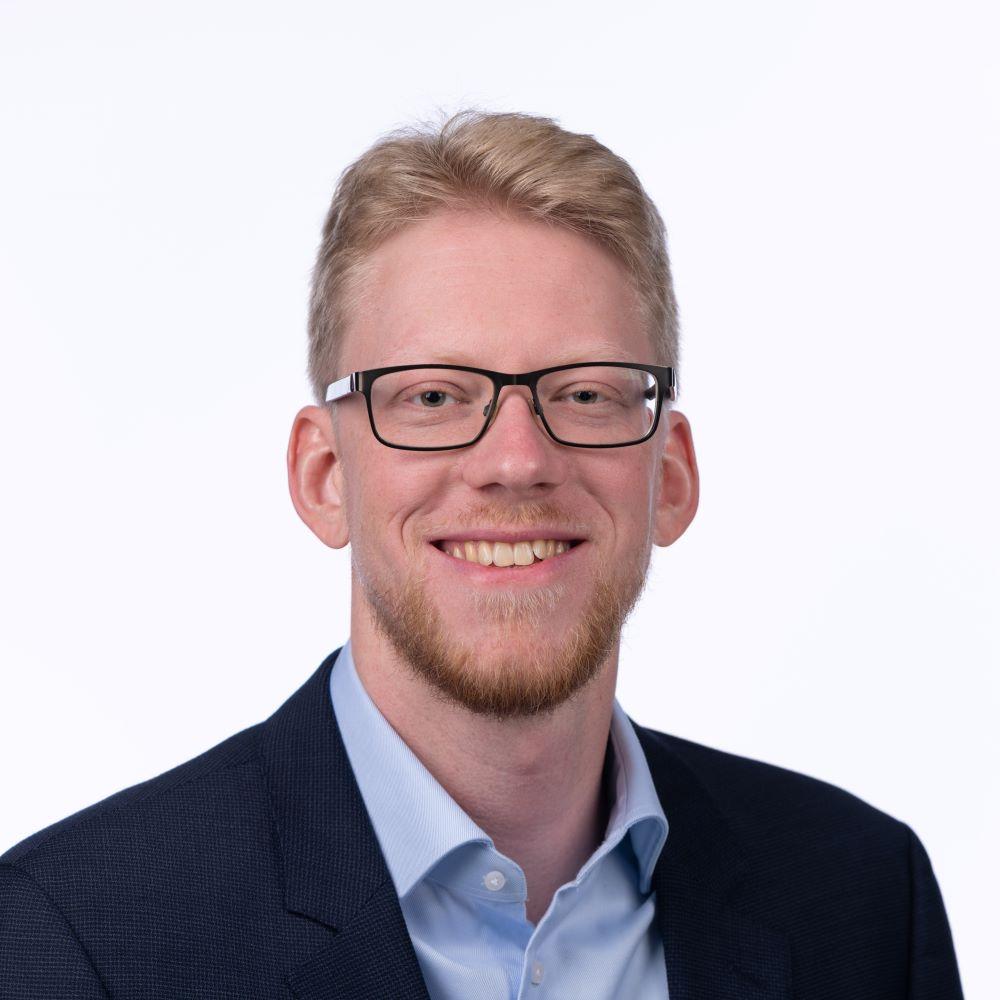
Leading AI4OPT's Operations, Research, and Future Directions
Abstract: As the managing director of AI4OPT, I oversee daily operations and lead a team of seven skilled individuals responsible for data security, partner relations, finances, event planning, communications, and more. Ensuring compliance with NSF standards is a key part of my role. I also stay involved in research, such as our project with Ryder on autonomous trucking. What I enjoy most is seeing collaboration among faculty and students, turning funding into impactful research, and organizing educational initiatives like the Faculty Training Program and Seth Bonder Camps. AI4OPT has grown into a mature organization with strong partnerships and impactful research. In the future, we plan to expand our team, develop new projects, and explore commercial applications of our work.
Biography: Kevin Dalmeijer is AI4OPT's Managing Director and a Senior Research Associate for the H. Milton Stewart School of Industrial and Systems Engineering (ISyE) at Georgia Tech. He obtained a Ph.D. in Operations Research from the Erasmus School of Economics, and he has worked on challenging problems in mobility, transportation, and logistics. His research focuses on redesigning public transit to improve mobility and automating supply chains to leverage AI and optimization.
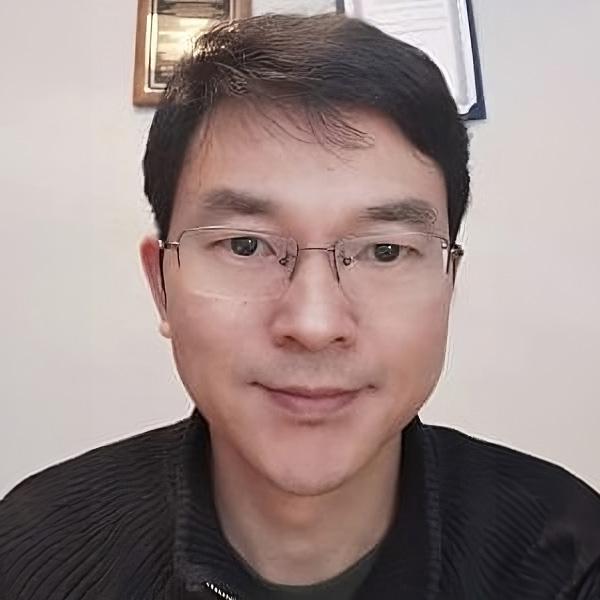
George Lan
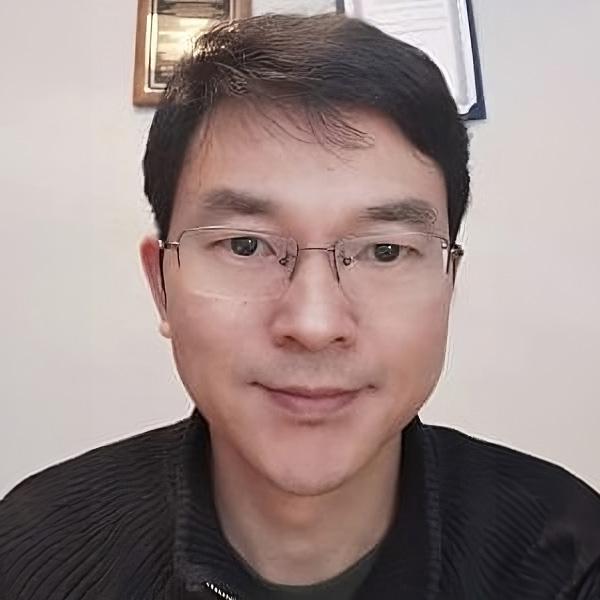
AI4OPT Decision Making under Uncertainty
Abstract: My work in the decision making under uncertainty methodology thrust includes a wide range of applications, such as sustainability, energy and manufacturing. Our primary goal is to make optimal decisions over a long period, even when the future is unknown. Collaborating with other AI4OPT thrusts is crucial to designing better process control systems that lead to the production of reliable renewable products. Currently, I am involved in three exciting projects that aim to advance the field of decision making under uncertainty.
Our first project focuses on developing stochastic optimization models and algorithms to solve stochastic problems over an infinite horizon. This involves developing algorithms that can handle a wide range of time horizons. Our team has developed a hierarchical multi-stage stochastic valuation model and algorithms to address these challenges, which is a significant advancement in our ability to tackle complex problems. The second project involves developing distributionally robust Bayesian optimization (DRBO). The goal is to create a new approach to address uncertainty in decision making, which is a common challenge in many applications. This approach involves considering the worst-case scenario while also considering the probability distribution of possible outcomes.
The third project is uncertainty quantification for multi-stage optimization. This project involves developing techniques to quantify uncertainty in multistage decision-making problems. The challenge lies in the fact that the uncertainty can evolve over time, and the impact of the uncertainty may change as the decision-making process unfolds.
Along with these projects, we explore the advantages of converting waste into a valuable resource in our work on "Sustainability: Waste to Treasure." The process offers several advantages over conventional waste disposal methods, including land conservation, pollution reduction, and renewable energy production. However, the process faces challenges in feedstock supply and composition, as well as complex and non-linear process dynamics.
In addition, my work with Intel focuses on the chip fabrication process flow, which involves the entire process from bare silicon wafer to fab (wafer processing) to e-test/sort to package assembly to component test to ship to customer.
With limitless applications for decision making under uncertainty, I am excited to continue this work and explore new solutions to long-standing problems. By having continuous collaborating with partners, we can broaden the range of our applications and help more industries benefit from this methodology.
Biography: Guanghui (George) Lan leads the AI4OPT methodology thrust: Decision Making under Uncertainty. He is also an A. Russell Chandler III Professor of Industrial and Systems Engineering at Georgia Tech. His research focuses on designing efficient algorithms for optimization problems in data analytics, machine learning, and reinforcement learning. Lan serves as an associate editor for three leading journals in the area of optimization. He is also an associate director for the Center of Machine Learning at Georgia Tech.
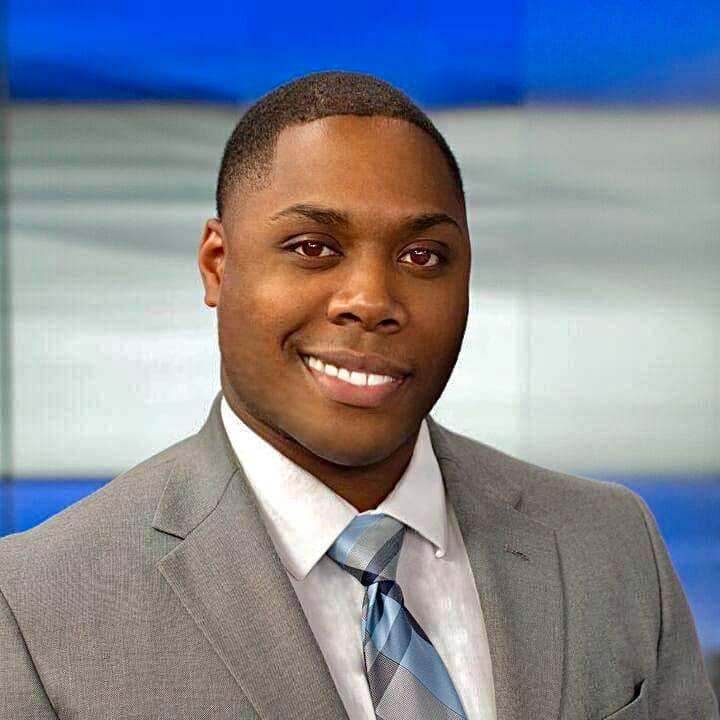
Breon Martin
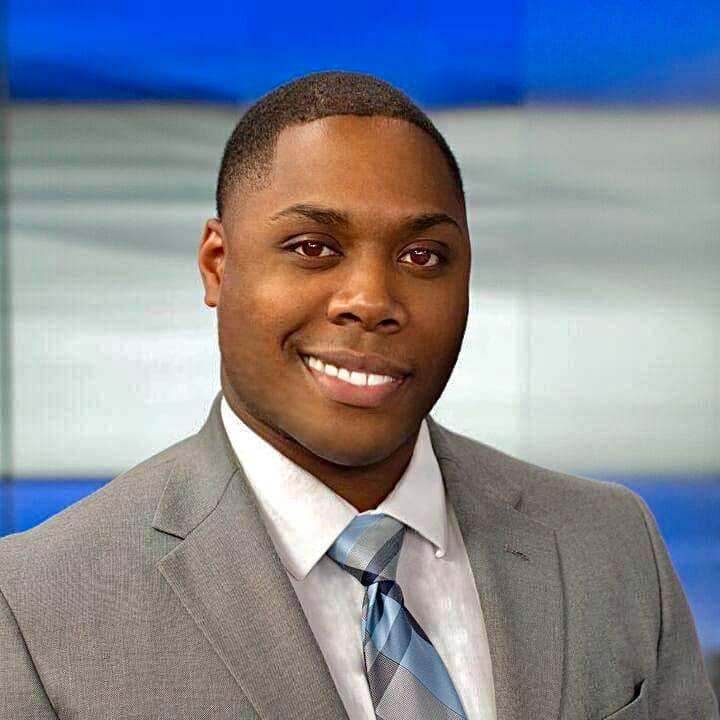
From Journalism to AI Communications
Abstract: I've always had a strong passion for journalism since college. My experience as a news anchor and reporter fueled my interest in covering technology and STEM. Transitioning to Communications at Georgia Tech felt like a natural step, allowing me to combine my writing skills with my love for technology. As an AI communications manager, I enjoy interviewing researchers and creating engaging content that showcases their work. Research topics like AI in healthcare and supply chain are particularly fulfilling. Working with the Institute Communications team has provided me with great resources to highlight the exceptional work of our members. Effective communication is integral to my role, and I take pride in making knowledge accessible and bringing out the personalities of those I interview.
Biography: Breon Martin, AI4OPT's Communications Director, brings over 7 years of TV news experience from New York, California, Louisiana, Kentucky, and Indiana, with multiple Emmy awards. As an AI Research Communications Manager, Breon also oversees communication efforts for AI-CARING and AI-ALOE institutes at Georgia Tech. He manages all communication aspects, with a focus on marketing and covering updates in AI and STEM education.
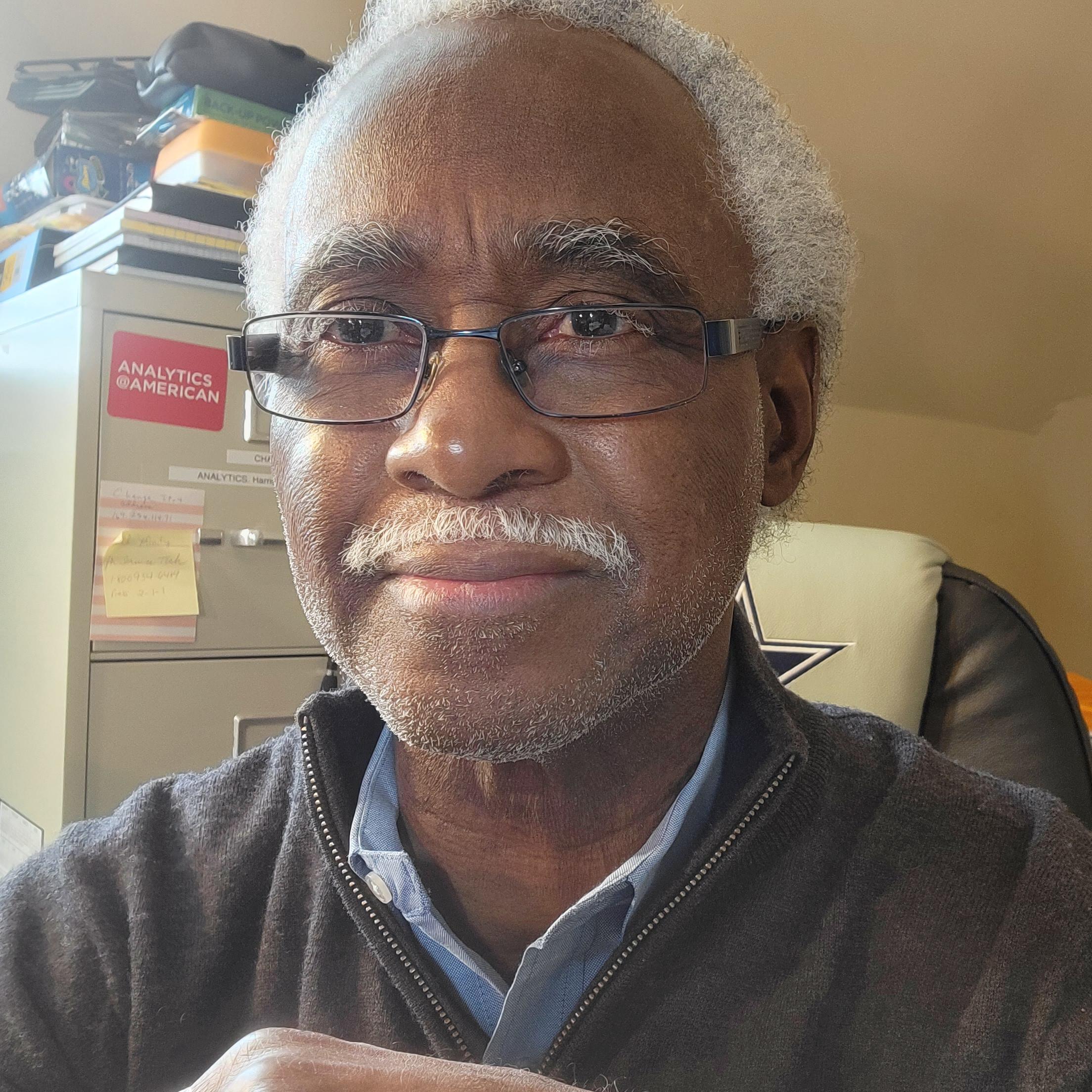
Charles Pierre
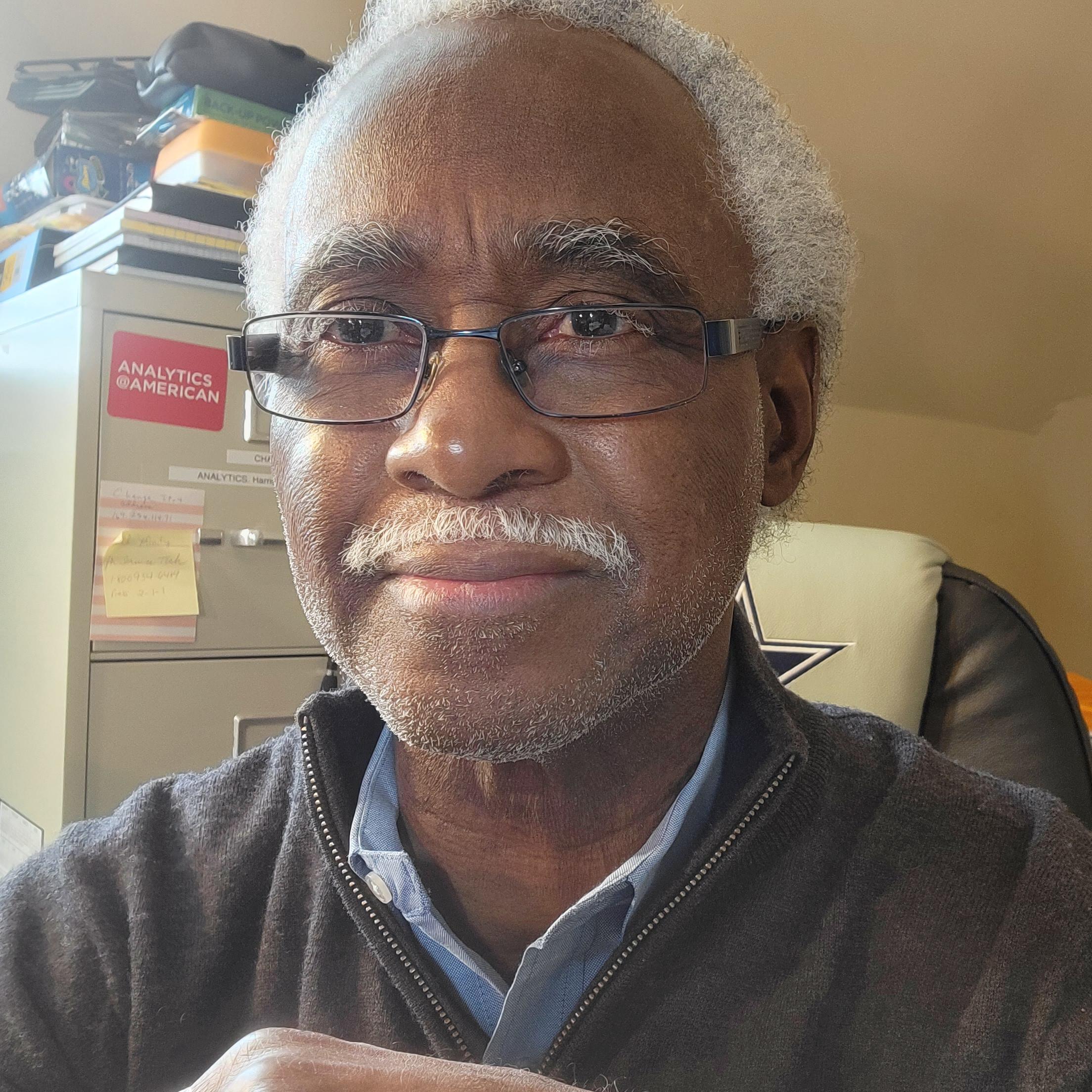
AI4OPT’s Educational and Diversity Thrust
Abstract: Supporting the growth of underrepresented populations in artificial intelligence (AI) is not only vital in the step for AI equity and inclusion, but also important to help empower future leaders and teachers across the world. We saw that there was a dearth of Black and Hispanic Americans in the field of AI— particularly in operations research and analytics and data sciences. AI4OPT has created strategies and programs to bolster and increase the numbers of underrepresented minorities in the field of AI.
Our Institute is using the “decision tree” approach whereby we train college and university professors, and university professors. This approach allows them to go back and train their respective undergraduate students; thereby, creating a pipeline for their students to enter the field of AI.
Last June, we introduced the first cohort of the AI4OPT Faculty Training Program. The program, held at Georgia Tech, consisted of faculty members from Historically Black Colleges and Universities (HBCU’s) and Minority Serving Institutions (MSI’s) from states including Georgia, California, Texas, and Virginia. The three-year program is comprised of courses in AI data science and course design.
In today’s ever-evolving higher education landscape, we see that schools are beginning to recognize the importance of investing in faculty training programs much like this one. Fundamentally, higher education faculty have built their careers around learning and teaching, so they understand the importance of continual education. For many of the instructors who participated in the program, they take it upon themselves to seek out learning opportunities and create ones if they are not provided the options or means at their institution.
The work we are doing is innovative, forward-thinking, and revolutionary. We aim to establish a minor or major data science program for each of the participants’ home institutions, as each participant will be equipped to set up joint research projects with AI4OPT researchers at Georgia Tech, UC Berkeley, and the University of Southern California to eventually host their own data science seminars and workshops.
Biography: Charles Pierre is the thrust leader for the educational and diversity initiatives of AI4OPT. Pierre is also an associate professor of mathematics at CAU and a 2020 INFORMS DEI Fellow.
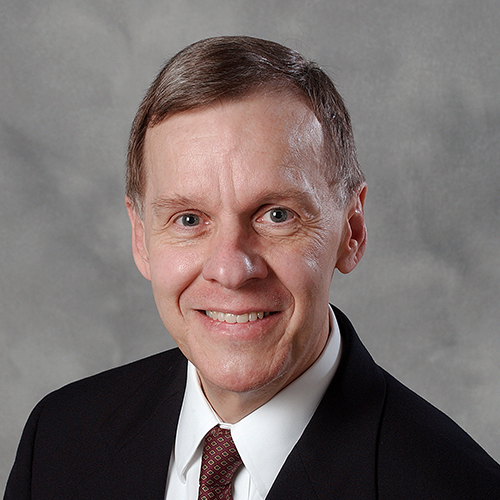
Chelsea White
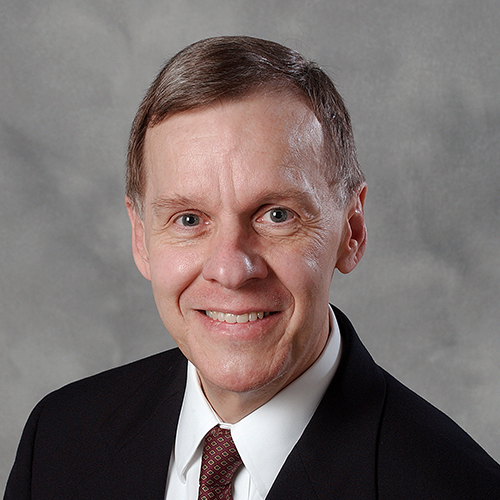
AI4OPT and Supply Chain Disruption, Visibility and Next Generation Supply Chain & Ecosystem Design
Abstract: Prior to the COVID-19 pandemic, risks in supply chains were typically local and of short duration, a perfect environment for lean supply chain design focused on cost reduction. In contrast, the pandemic has had long-term impact on everything from suppliers to manufacturing to distribution, and has caused a significant increase in demand volatility. Right now, the worst kind of design for supply chains - if you’re going to be faced with disruptions - is a lean supply chain. With no resilience built-in, no buffer stock, and no buffer manufacturing capacity, lean supply chains don’t hold up well when faced with disruptions.
Considering this new trade environment, global supply chains have had to adjust from lean to more expensive agile supply chains that have contributed to inflation and have raised questions like: How do we now balance risks of disruptions in supply chains versus the reduction of costs? We are working toward supply chain design solutions as we face the lingering effects of Covid-19, as well as the recent increase in extreme weather events and geopolitical risk, and a way of better aligning private sector and public sector interests when faced with disruptions that can affect the United States public health, defense, or economic security. This alignment is a significant challenge as large companies typically do not share the same devices, platforms, objectives, or data among themselves or with the public sector.
A current interest of mine is to develop a stress test process to better understand how we can find the right balance of risk and cost for a company in an environment of increased volatility. My colleagues and I are being supported by two Manufacturing Innovation Institutes, BioMADE and ARM (Advanced Robotics for Manufacturing), to develop and test such a process. Through BioMADE, we are collecting data from a large multinational corporation and a startup company. Through ARM, we are working with a small to medium size enterprise that makes oxygen concentrators to better understand how disruptions can affect their supply chain performance and how best to mitigate disruption risks.
Biography: The research of Prof. Chelsea C. White has been driven by the challenges faced by U. S. supply chains since the start of the pandemic from both private and public perspectives.
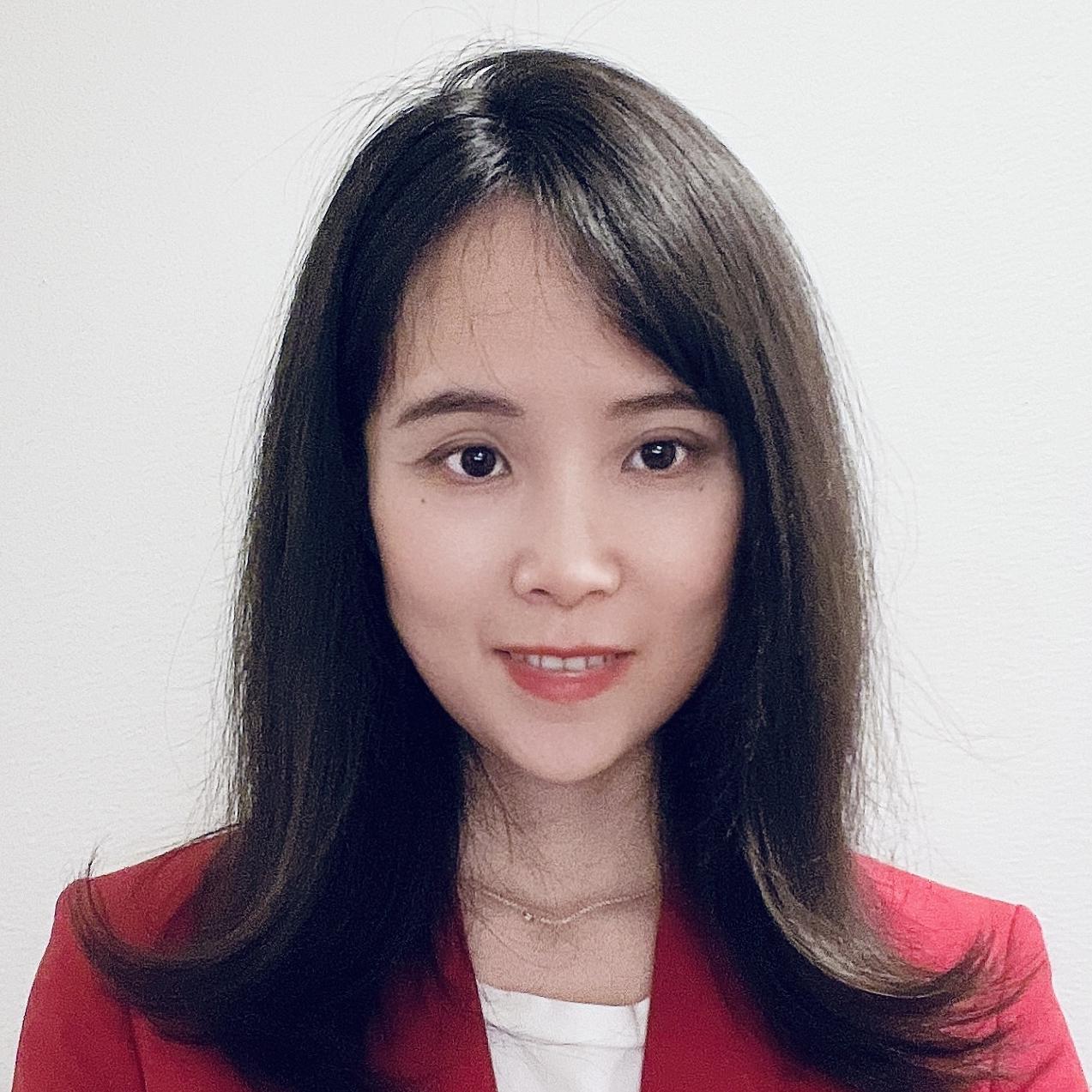
Yao Xie
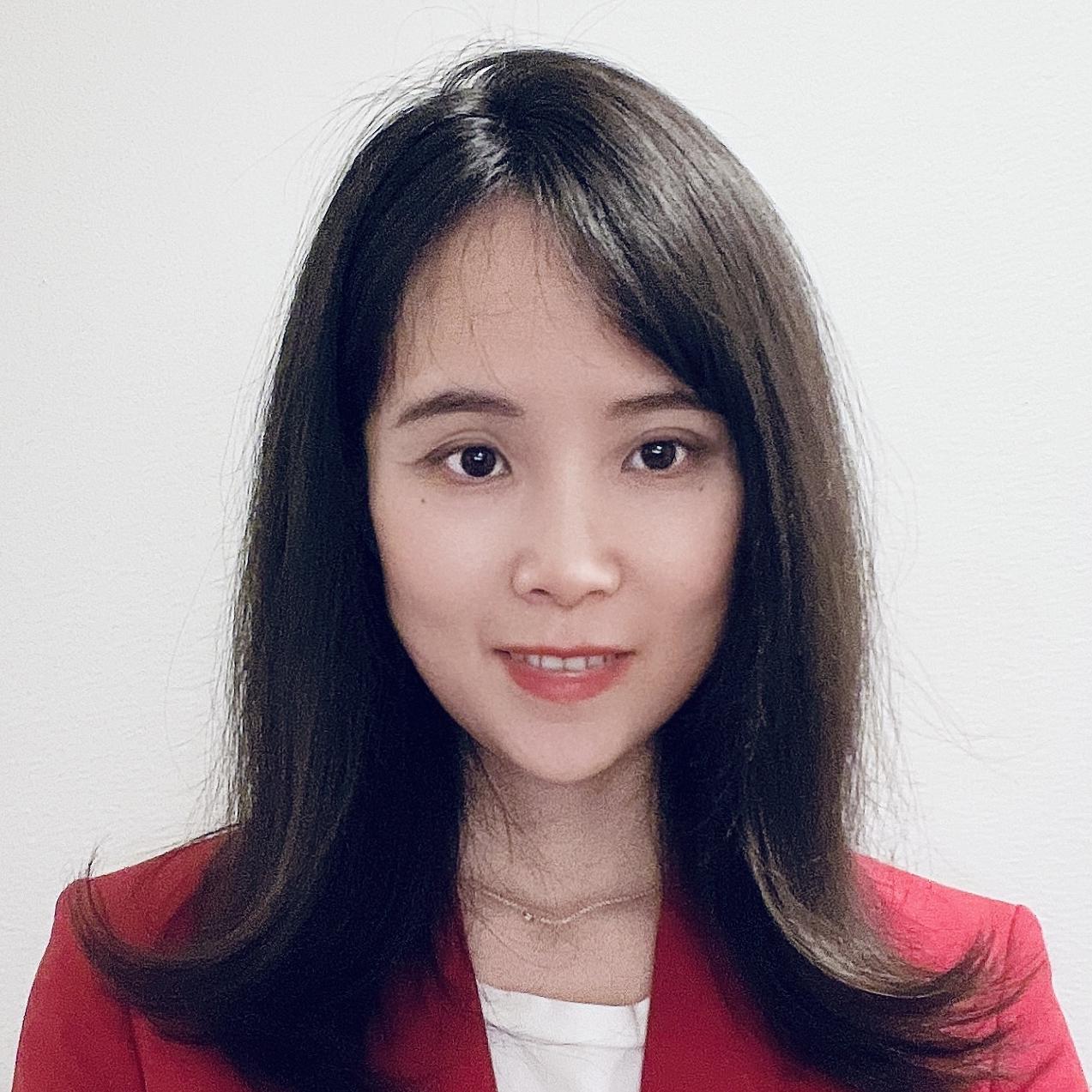
Uncertainty Quantification Using Conformal Inference and Neural Networks for Prediction
Abstract: Uncertainty quantification (UQ) has proven to be increasingly valuable in prediction pipelines and is closely related to both AI4OPT’s methodology and theory developments. My primary research area is in statistics, particularly change-point detection in sequential and spatio-temporal data, and hypothesis testing. These efforts under AI4OPT’s decision-making and uncertainty thrust have a direct effect to energy use systems.
We want our spatio-temporal prediction (STP), which is used in data analysis when data is collected across both space and time, to be used for wind power generation prediction and solar power generation prediction. We are using novel methods based on conformal prediction, called CONFOR-MAL, which is a new type of distribution free methods for quantifying the uncertainty for general machine learning predictive methods.
The wind power generation data we have is from a wind farm in the Midwestern United States region. The challenge is that the data is time varying. Winds not only changing in magnitude but also direction which can leave the prediction to become more complex when you take both time variations into account. We also need to do this, considering the spatial temporal correlations of the data and a lot of the predictions in the past have been one dimensional times series.
Now we're considering multidimensional and high dimensional time series where each dimension of the time series corresponds to one location, wind power generation and the different directions. We want to do the prediction for spatio-temporal setting because in these use cases such as wind power predictions, we need to consider prediction at the same time for different locations. So, the prediction is based on graphs in certain networks.
Harvesting neural network-based methods coupled with conformal inference to quantify the uncertainty of the prediction is also important for the next stage of optimization algorithms.
Biography: Yao Xie is an Associate Professor and Harold R. and Mary Anne Nash Early Career Professor at Georgia Institute of Technology in the H. Milton Stewart School of Industrial and Systems Engineering, and an Associate Director of the Machine Learning Center. She received her Ph.D. in Electrical Engineering (minor in Mathematics) from Stanford University and was a Research Scientist at Duke University. She received the National Science Foundation (NSF) CAREER Award in 2017, INFORMS Wagner Prize Finalist in 2021, and the INFORMS Gaver Early Career Award for Excellence in Operations Research in 2022. She is currently an Associate Editor for IEEE Transactions on Information Theory, IEEE Transactions on Signal Processing, Sequential Analysis: Design Methods and Applications, INFORMS Journal on Data Science, and serves on the Editorial Board of the Journal of Machine Learning Research, and an Area Chair of NeurIPS in 2021 and 2022.
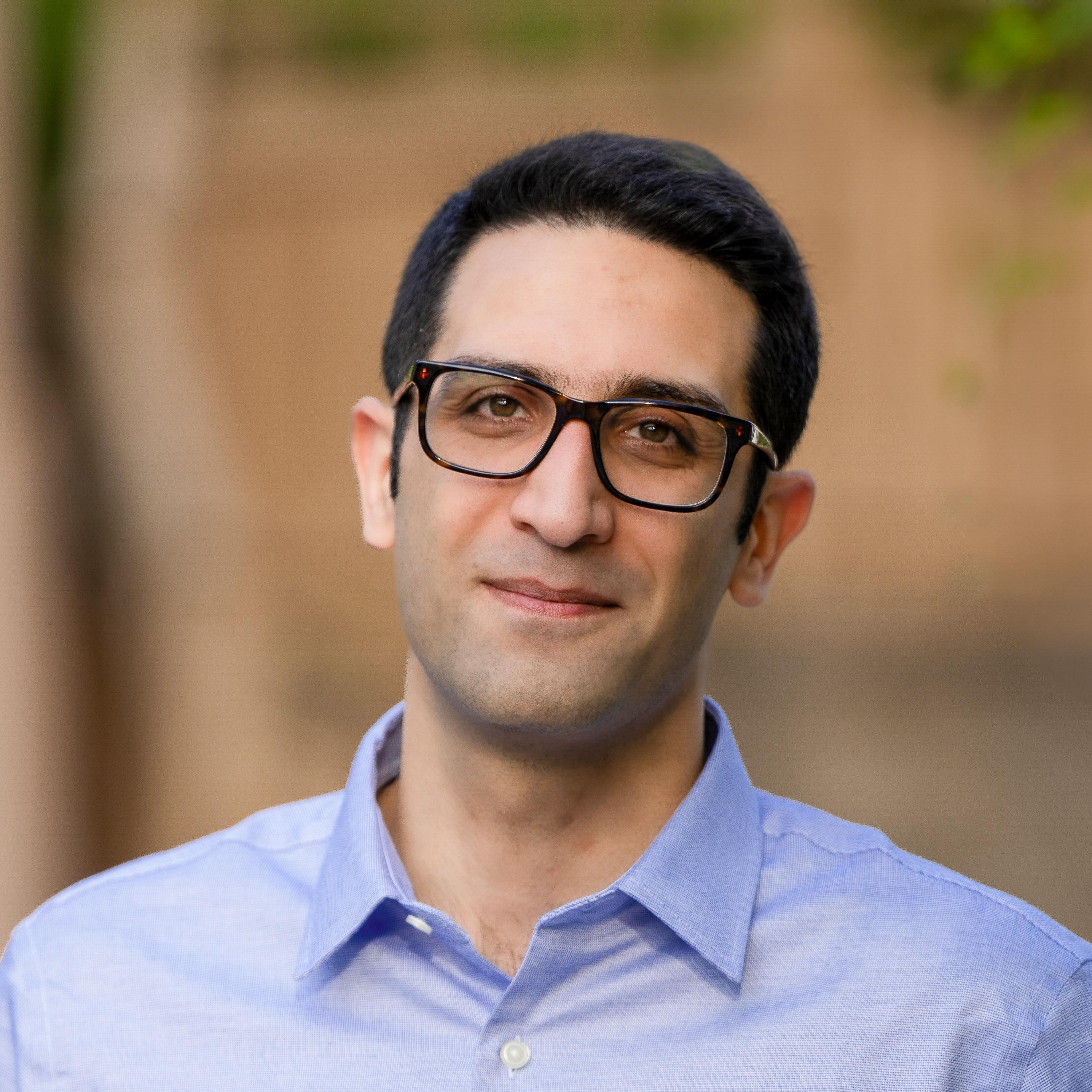
Reza Zandehshahvar
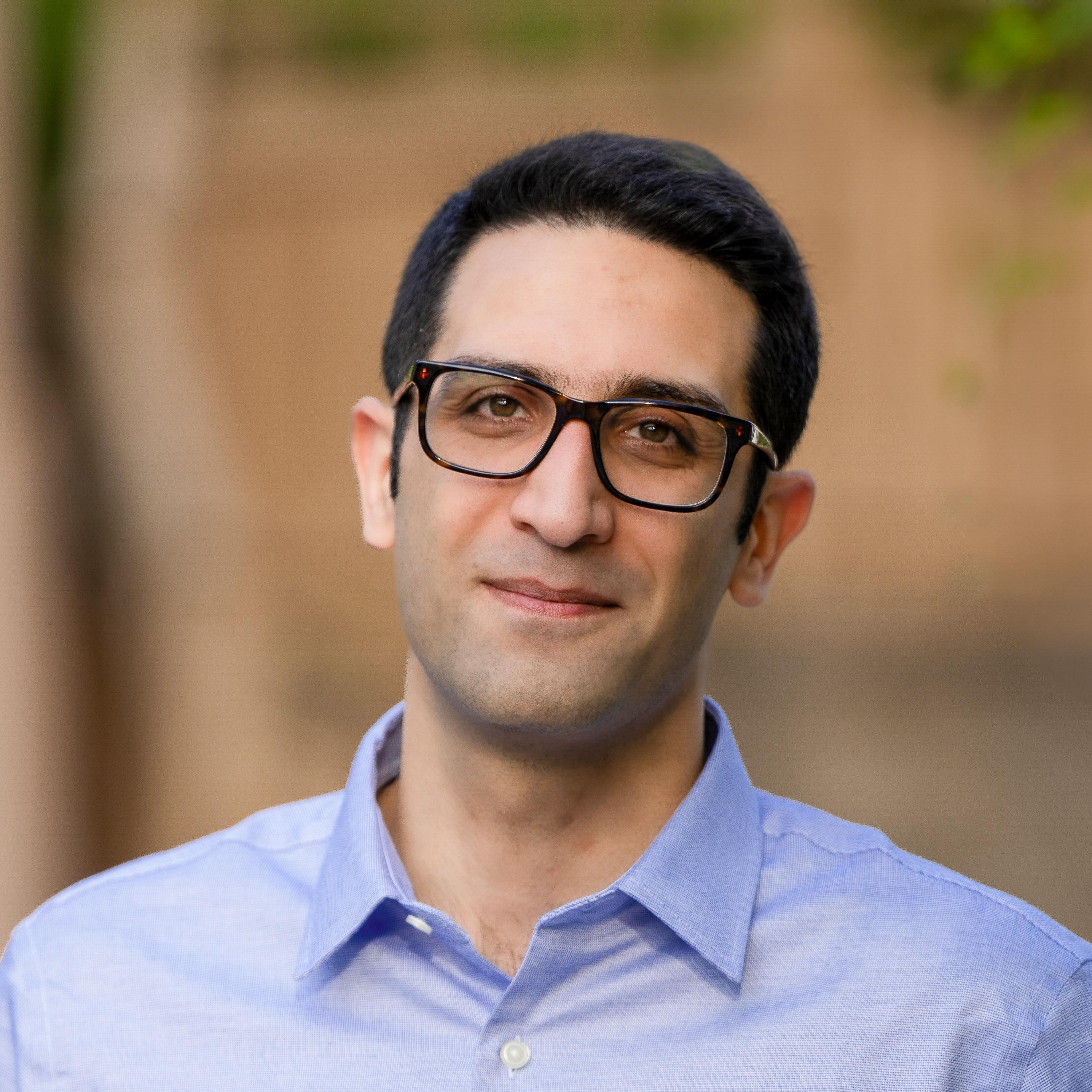
Advancing Trustworthy AI for Decision-Making in Healthcare, Supply Chain, and Power Systems
Abstract: My research focuses on developing trustworthy AI frameworks by integrating machine learning with optimization techniques. The goal is to create robust, explainable, and adaptable models for decision-making in high-stakes environments such as healthcare, supply chain management, and power systems. Collaborating closely with students and fellow researchers, we push the boundaries of practical AI applications.
My foundational work involves developing uncertainty quantification techniques crucial for sensitive fields like healthcare, supply chain management, and power systems, where decisions significantly impact people's lives. This includes creating AI-assisted decision-making tools for package delivery, planning in supply chain management, and confidence-aware time series prediction.
In healthcare, my work at AI4OPT includes a collaboration with Shriners Children's Hospital to improve treatment strategies for children with idiopathic scoliosis. By developing predictive models for children's growth rates and growth cessation using various data sources, we aim to enhance prognosis strategies and tailor treatments according to individual growth trajectories, ultimately improving overall patient outcomes. Ensuring the explainability and trustworthiness of these models is essential.
Biography: Reza Zandehshahvar is a postdoctoral fellow with the NSF Artificial Intelligence Institute for Advances in Optimization (AI4OPT) at the H. Milton Stewart School of Industrial and Systems Engineering at Georgia Tech. He earned his Ph.D. in electrical and computer engineering from Georgia Tech in 2023, focusing on developing machine learning methods for inverse design and knowledge discovery in nanophotonics, as well as enhancing human-machine cooperation for medical image analysis. Zandehshahvar has received awards such as the Georgia Tech Sigma Xi Best Ph.D. Thesis Award, the SPIE Optics and Photonics Education Scholarship, and the Georgia Tech ECE Graduate Research Assistant Excellence Award. In his current role at AI4OPT, under the supervision of Professor Pascal Van Hentenryck, he is focused on developing trustworthy AI methods that enable reliable and efficient decision-making within the engineering and healthcare sectors.